Abhejit Rajagopal, PhD Spotlight
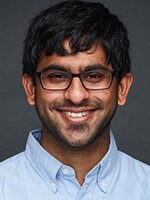
September 23, 2022
Abhejit Rajagopal, PhD, is a Postdoctoral Fellow in the Department of Radiology & Biomedical Imaging and an expert in designing robust machine learning algorithms for image reconstruction and classification. An engineer by training, Abhejit is a deep learning generalist and approaches medical imaging with the same relentless fascination and sensitivity for potential consequence that he applied in his dissertation research on high resolution systems for military drones. He sees incredible potential for incorporating physics into the way we do machine learning. His goal is to understand the neural networks that process images to the point that we can predict when they are going to fail.
Abhejit feels like he’s been in the University of California for his entire life. He grew up in Irvine right near the UC campus and volunteered in the UCI physics lab during high school. He received his BS in Electrical Engineering from UCLA where he worked on signal processing and circuits, and then moved up the coast to UC Santa Barbara for both an MS and PhD in Electrical and Computer Engineering where he focused on applied mathematics and the physical basis of imaging. Now, he has continued north and joined UCSF to expand his research on the medical applications of machine learning and neural nets. When asked what question guides his research, Abhejit said, “I want to answer, ‘How do you measure the world?’”
Rajagopal, Abhejit, et al. "Enhanced PET/MRI reconstruction via dichromatic interpolation of domain-translated zero-dose PET."
Medical Imaging 2021: Physics of Medical Imaging. Vol. 11595. SPIE, 2021.
He is an engineer by training, but through his study of machine learning, analyzing imaging became his passion and from there he ventured into radiology. While in grad school at UCSB he contacted UCSF to “sneak” his way into the radiology research seminar despite admittedly not being a radiologist or having any medical training. He presented his poster “Deep iterative-reconstruction algorithms,” a physics-based approach to designing algorithms for neural network image reconstruction. Drawn by that presentation, Peder Larson, PhD, connected with him and ended up offering the engineer presenter a postdoc position. Soon after, he met with Tom Hope, MD, and with them as his mentors Abhejit’s work at UCSF began.
Reflecting on his time at UCSF, Abhejit observed that, “UCSF is an elevated place, a very different world from engineering school. I was used to working on problems on the board or otherwise performing really far away from the actual medical applications, but at UCSF everyone is interested in doing science. Working with Tom Hope and Peder Larson has been amazing. I never thought I would get a job where I get to do what I love to do, all the time.”
Abhejit is a proponent of using AI in medicine and believes the technology is ready, but we as the human element still need to practice how to use it effectively. “AI is ripe for supplementing doctors’ abilities rather than replacing them. Patients get the benefit of better diagnoses informed by hundreds of thousands of cases. This kind of data has always been shared through medical journals and other publications or through doctors in discourse with other doctors, but now we have the means to share data on a massive scale and accelerate that process of group learning.”
Abhejit approaches expanding the capabilities of neural training and imaging from a perspective of raw mathematics and physics. “We usually think of machine learning as a black box,” he said, “where you provide some input and want to get some predictions out. We want the process of turning inputs into predictions to incorporate explicit knowledge about the world. The structure of the neural network should be influenced by the physics of the problem, for example in PET physics; how positrons recombine, and how they emit photons, how they travel and how they are measured, how the collector then digitizes that information. The neural network needs to incorporate an understanding of that entire physical system and structurally enforce that understanding to produce trustworthy predictions.”
Rajagopal, Abhejit, et al. "Understanding and Visualizing Generalization in UNets." Medical Imaging with Deep Learning. PMLR, 2021.
His goal is to perfect a “neural trace” that can understand the ways a neural network arrives ats its conclusions and thus predict when such a system may fail. In medicine, there are gray borders around such conclusions, such as the definition of clinically significant cancer. Neural networks, like radiologists, can sometimes struggle to determine what is clinically significant cancer just from an MRI. However, that struggle or uncertainty is not always clearly visible in the AI’s output. An AI will return a result but, “How do you know when the AI is working well or not? We need a program to tell the doctor receiving the images how confident the network is in its own extrapolations. Using this “neural trace” will identify if the neural network is able to classify this image correctly or if it is more likely to make a prediction that it is not really confident in. The goal is to have a model that can piggyback on any system and tell the doctor the degree of confidence with which the AI is making its prediction.”
UCSF has proved to be a wonderful playground of discovery and collaboration. Reflecting on this period of his career, Abhejit notes that “I was surprised by just how supportive my mentors have been. I have always had diverse research interests, but at UCSF with the support of Peder and Tom I’ve had the encouragement, resources, and critical feedback necessary to translate my interests into discoveries. The coolest thing about our field is we’re able to see our ideas come to life in pictures, and it is so incredibly rewarding.”
Having had the fortune to benefit from great mentors himself, Abhejit places a high priority on mentoring and teaching. The Center for Intelligent Imaging launched its education pillar a couple years ago and he gave the intro to machine learning for that very first class. “Teaching not only clarifies our own understanding, but helps train the next generation of scientists, who will be the ones to carry out work with the infrastructure we are designing now. Our success really depends on their success.”
Even with advances in how machine learning algorithms process images and the promise of his “neural trace”, Abhejit acknowledges that current practices such as surgical biopsies for cancer are not yet due to become a thing of the past. “That has always been the hope. But it really comes down to ‘What would you choose for your own diagnosis?’ Wouldn’t you want to still have a biopsy, just to be sure? Instead of replacing biopsies, AI is ripe for complementing current clinical practice, for example by informing where exactly to perform the biopsy, or by providing additional insight into the spatial distribution of cancer. By combining the imaging AI and the procedure, you can be doubly sure.”
However, Abhejit is at his heart a technological optimist. An ever eager generalist, Abhejit always has one eye on the horizon for the future of new breakthrough image systems in many fields. The sudden rush of strangely hypnotic AI generated artwork from programs such as DALL·E that have swept into popular view over the past year have inspired Abhejit with their potential. These text-based image transformation systems have many medical implications. “Leveraging text-based imaging for clinical diagnosis and comparison would have amazing impacts. If we could jointly interpret text from physician notes with the imaging results inside the same algorithm, we could increase AI training dataset sizes by 100 to 1000 times, which has the potential dramatically improve AI model generalization performance, especially for rare diseases.”
Another game changer, and one that Abhejit is personally part of, is the advancement of federated learning. Machine learning has been hampered by the licensing and legal hurdles that usually lead to each institution training their own models. A federated system allows multiple institutions to work together to train one algorithm and improve over what is possible at any one site. Abhejit and others have started a cooperation program with UCLA and very soon federated.ucsf.edu will be open for collaboration with other UCs and research institutions. “You have to make this technology easy for people to use. Big companies like Google and Amazon have been able to scale these extremely large systems for years, but making this accessible to researchers has the potential to revolutionize how we train, validate, and deploy radiology AI.”
Abhejit first moved to San Francisco early in 2019, and is currently working remotely due to the COVID pandemic. He loves hiking with his partner and his rambunctious husky, who is a source of joy but certainly a handful. However, those four miles a day of dog-walks are an important way to avoid the threat of burn-out. “I’m my best when I’m feeling connected with nature. I find that walking outside, often aimlessly, is where I do my best thinking. I have solved so many problems that way, it’s part of my process.”