A Domain Adaptation framework for Neural Network based Medical Image Segmentation
Date
Type
Time Duration
Location
Speakers
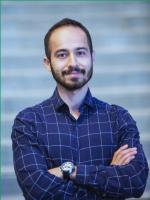
Berkeley Institute for Data Science (BIDS)
Abstract: I will present a new segmentation framework that uses deep neural networks which involves two innovations. First, I will describe a biophysics-based domain adaptation method. Second, in addition to tumorous tissues, we propose an automatic method to segment white and gray matter, and cerebrospinal fluid. Regarding our first innovation, we use a domain adaptation framework that combines a multispecies biophysical tumor growth model with a generative adversarial model to create realistic looking synthetic multimodal MR images with known segmentation. Regarding our second innovation, we propose an automatic approach to enrich available segmentation data by computing the segmentation for healthy tissues. This is performed through a novel diffeomorphic image registration between the training data and a set of prelabeled atlases. This provides more information for training and reduces the class imbalance problem. Our overall approach is not specific to any particular neural network and can be used in conjunction with existing solutions. We demonstrate the performance improvement on BraTS segmentation challenge, showing a significant 5% higher whole tumor dice score as compared to state-of-the-art GAN proposed in the literature.